Insights / Articles
OPUF: Getting Personal About Preferences
Written by Marieke Heisen on Friday, October 21, 2022
There is growing recognition that patient preferences matter. Traditional preference elicitation techniques, such as the discrete choice experiment (DCE) and time trade-off (TTO) method, are not always well suited to obtaining patient preferences, as they rely on large sample sizes to derive average preferences, also called a value set, value function, or utility function. With these techniques, a value set is the result of unravelling, or decomposing, the building blocks (attributes or dimensions) of value, by analyzing how much respondents’ choices (trade-offs) are driven by these attributes.
In some diseases — for example, rare diseases — large sample sizes are not feasible, making it difficult to use conventional methods. In this article, we explore a new online tool for eliciting personal health state utilities that is particularly well suited to smaller samples and has the ability to capture the preferences of individual patients.
It is the personal utility function (PUF) methodology, developed by Nancy Devlin, Ben van Hout, and colleagues to provide a robust way for individuals to value health states or treatment profiles. The approach draws on the theory and methods of multicriteria decision analysis. Paul Schneider transformed the pen-and-paper PUF into the online PUF (OPUF) and has researched its applicability and performance. (You can try out a demo version of the OPUF applied to the EQ-5D-5L here.)
OPUF is useful for small samples, as individual utility functions are gathered. The individual respondent undertakes a series of exercises to build – compose – their own utility function. Such a compositional technique permits access to rich information at the individual level and can also provide insights on the aggregate level. The exercises facilitate deliberation – e.g., respondents need to ask themselves: What attribute do I find most important and how much more important is it to me than the others? Respondents can also confirm or adjust their preferences when the resulting utility function is shown to them.
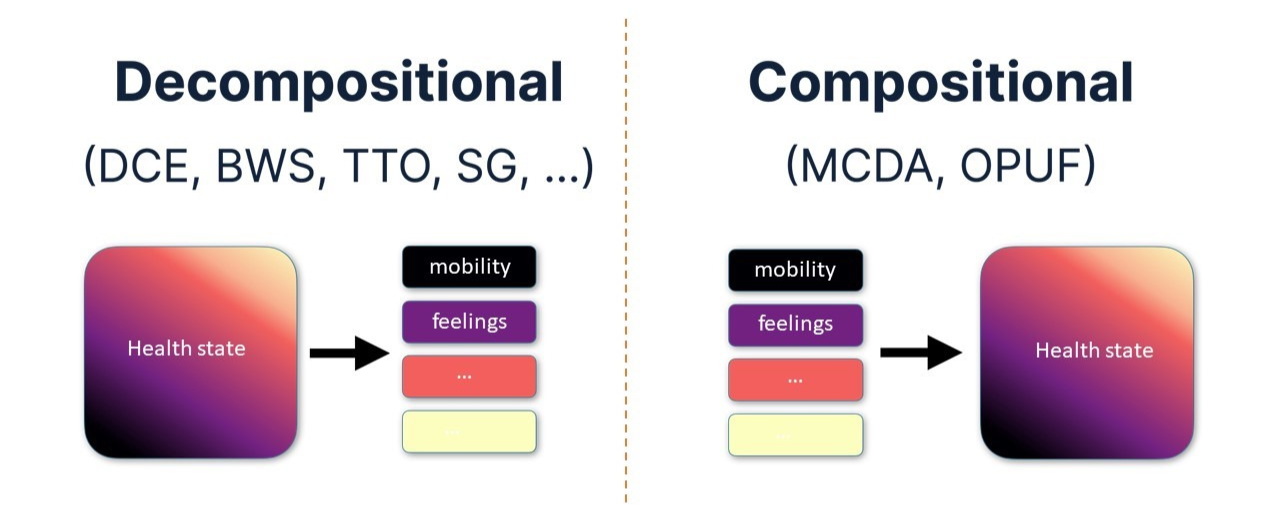
OPUF is useful with small samples, but its ease and speed of use make it suitable for larger samples, too, such as the kind of general population samples used to construct value sets for generic HRQoL instruments. It has particular strengths compared with conventional methods when there is specific interest in preference heterogeneity, when there are many attributes and levels, or when there is interest in following preferences over time.
JOIN US
Visit our careers page to view opportunities at OPEN Health.
CAN WE HELP?
For information about OPEN Health’s services and how we could support you, please get in touch.